Debezium Blog
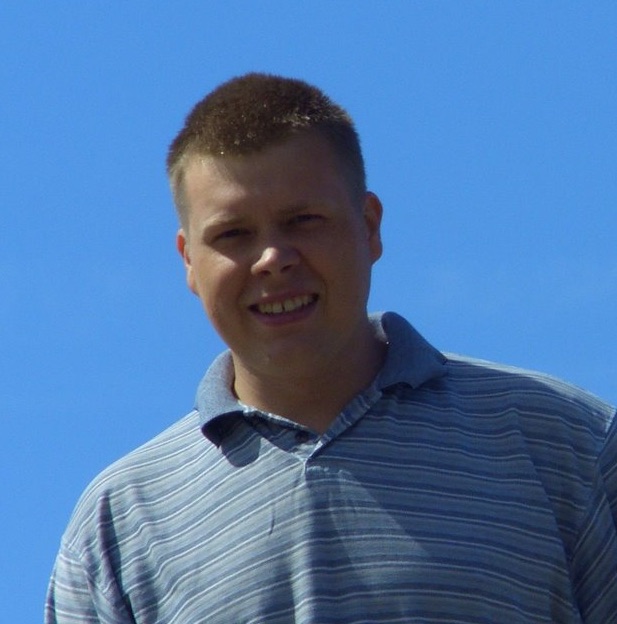
As a follow up to the recent Building Audit Logs with Change Data Capture and Stream Processing blog post, we’d like to extend the example with admin features to make it possible to capture and fix any missing transactional data.
In the above mentioned blog post, there is a log enricher service used to combine data inserted or updated in the Vegetable database table with transaction context data such as
-
Transaction id
-
User name who performed the work
-
Use case that was behind the actual change e.g. "CREATE VEGETABLE"
This all works well as long as all the changes are done via the vegetable service. But is this always the case?
What about maintenance activities or migration scripts executed directly on the database level? There are still a lot of such activities going on, either on purpose or because that is our old habits we are trying to change…
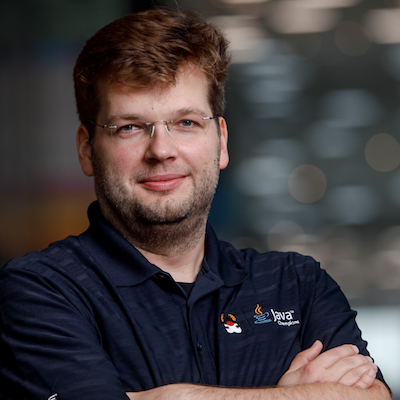
It is a common requirement for business applications to maintain some form of audit log, i.e. a persistent trail of all the changes to the application’s data. If you squint a bit, a Kafka topic with Debezium data change events is quite similar to that: sourced from database transaction logs, it describes all the changes to the records of an application. What’s missing though is some metadata: why, when and by whom was the data changed? In this post we’re going to explore how that metadata can be provided and exposed via change data capture (CDC), and how stream processing can be used to enrich the actual data change events with such metadata.
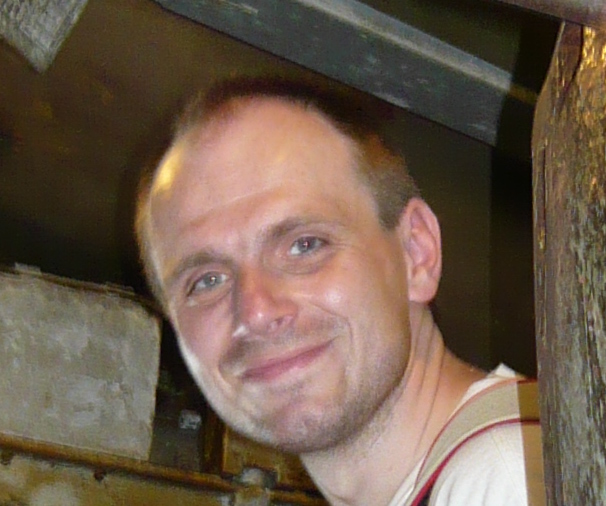
Last week’s announcement of Quarkus sparked a great amount of interest in the Java community: crafted from the best of breed Java libraries and standards, it allows to build Kubernetes-native applications based on GraalVM & OpenJDK HotSpot. In this blog post we are going to demonstrate how a Quarkus-based microservice can consume Debezium’s data change events via Apache Kafka. For that purpose, we’ll see what it takes to convert the shipment microservice from our recent post about the outbox pattern into Quarkus-based service.
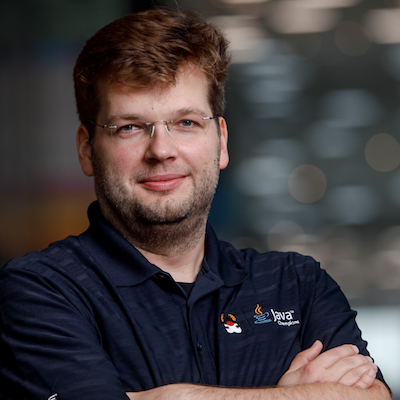
As part of their business logic, microservices often do not only have to update their own local data store, but they also need to notify other services about data changes that happened. The outbox pattern describes an approach for letting services execute these two tasks in a safe and consistent manner; it provides source services with instant "read your own writes" semantics, while offering reliable, eventually consistent data exchange across service boundaries.